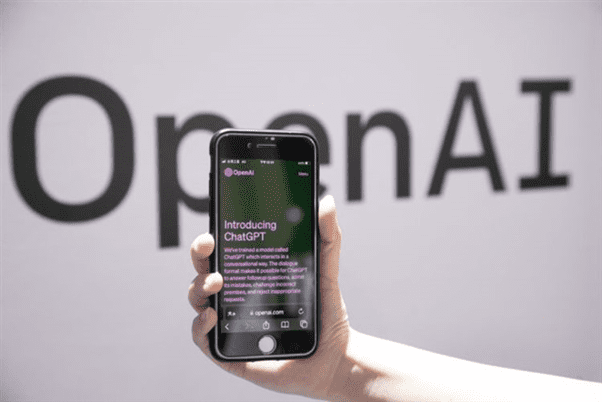
Google Trends is a useful tool to observe how trends are changing for specific topics. This time, I entered the three phrases that represent paradigm shifts in AI technology: “Expert System,” “Deep Learning,” and “Generative AI” to observe the results.
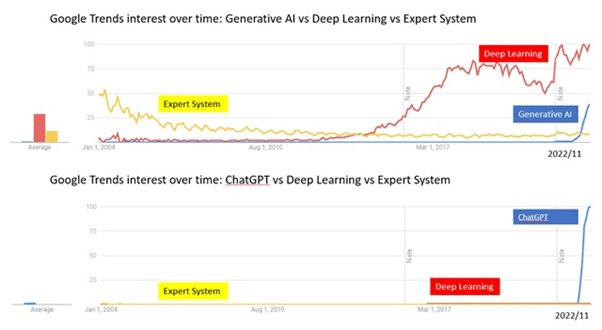
Using 2004, the earliest available data point in Google Trends, as a starting point, interest in expert systems has continued to go down. In contrast, interest in deep learning started to rise in 2013 and surpassed expert system in 2014, while generative AI experienced a significant jump when ChatGPT was unveiled in November 2022.
Expert systems are a true commercialized AI technology in the early days and belong to rule-based learning. An expert system consists of three components: knowledge base, inference engine, and user interface.
Knowledge bases are built by consulting a large number of experts and then using an if-then-else structure to feed the experts’ knowledge and experience into the base. The inference engine makes inferences and decisions based on the rules and mechanisms within the knowledge base. The user interface acts like ChatGPT, allowing users to obtain the answer inferred by the expert system in the form of a Q&A.
The expert systems trend started to decline in the 1980s due to the difficulty of expressing and forming rules for much of humans’ hidden knowledge, as well as the increasing complexity of rebuilding and maintaining rule-based learning databases. Expert systems in professional fields (e.g., medical and civil engineering) gradually died out, while rule-based systems for general business management were gradually integrated into corporate application software from companies such as Oracle and SAP.
In 2012, University of Toronto professor Geoffrey Hinton and his two doctorate students Alex Krizhevsky and Ilya Sutskever announced the paper “ImageNet Classification with Deep Convolutional Networks,” which gave rise to deep learning. In the same year, at the ImageNet Large Scale Visual Recognition Challenge (ILSVRC) initiated by Fei-Fei Li, their CNN (convolutional neural network) architecture AlexNet achieved a record-breaking error rate of 15.3% – nearly 11 percentage points better than the runner-up (26.2%).
Since then, deep learning has become the mainstream technology when it comes to machine vision. When Microsoft’s ResNet outperformed the 5% error rate of the human eye with an error rate of 3.6% in 2015, various market opportunities such as smart transportation, facial recognition, and defect inspection took off. This has been reflected in the search interests in deep learning since 2013.
Compared to deep learning, which performs recognition tasks like classifying and categorizing existing data (e.g., face recognition), generative AI learns the pattern and structure of the inputted data and then generates similar but new data based on the distribution of the training datasets.
The GAN (generative adversarial network) proposed by Ian Goodfellow in 2014 was a milestone in the development of generative AI. In the following years, the search interest in generative AI saw slight increases and began to receive more and more attention in professional communities. Then, the introduction of ChatGPT in November 2022 triggered the media and the general public’s attention and subsequent use, which led to a rapid increase in search interests.
If we directly compare ChatGPT to expert systems and deep learning in Google Trends, we can see that due to the explosive search interest in ChatGPT, the search interest for expert systems and deep learning has been reduced to a nearly flat line at 0.
In a 2007 oral history interview with the Computer History Museum, Edward Feigenbaum, the father of expert systems, responded to a question about AI and knowledge with the following: “There are several significant ways in which we differ from animals, but one way is that we transmit our knowledge via cultural mechanisms called texts. (It) used to be manuscripts, then it was printed texts, now it’s electronic texts… We need to have a way in which computers can read books on chemistry and learn chemistry, read books on physics and learn physics, or biology… Everything is hand-crafted. Everything is knowledge engineering.” (Excerpted from Oral History of Edward Feigenbaum at computerhistory.org)
Compared to deep learning, which performs recognition tasks like classifying and categorizing existing data (e.g., face recognition), generative AI learns the pattern and structure of the inputted data and then generates similar but new data based on the distribution of the training datasets.
The GAN (generative adversarial network) proposed by Ian Goodfellow in 2014 was a milestone in the development of generative AI. In the following years, the search interest in generative AI saw slight increases and began to receive more and more attention in professional communities. Then, the introduction of ChatGPT in November 2022 triggered the media and the general public’s attention and subsequent use, which led to a rapid increase in search interests.
If we directly compare ChatGPT to expert systems and deep learning in Google Trends, we can see that due to the explosive search interest in ChatGPT, the search interest for expert systems and deep learning has been reduced to a nearly flat line at 0.
In a 2007 oral history interview with the Computer History Museum, Edward Feigenbaum, the father of expert systems, responded to a question about AI and knowledge with the following: “There are several significant ways in which we differ from animals, but one way is that we transmit our knowledge via cultural mechanisms called texts. (It) used to be manuscripts, then it was printed texts, now it’s electronic texts… We need to have a way in which computers can read books on chemistry and learn chemistry, read books on physics and learn physics, or biology… Everything is hand-crafted. Everything is knowledge engineering.” (Excerpted from Oral History of Edward Feigenbaum at computerhistory.org)
Feigenbaum simply could not have anticipated at the time the incredible quantitative growth in training text due to developments in LLM made by the likes of Google’s BERT and OpenAI’s GPT. In OpenAI’s case, in 2018, GPT-1 had 5GB of training data with 110 million parameters. In 2020, GPT-3 had 45TB of training data with 175 billion parameters. Since then, OpenAI has stopped publishing its training data volumes, but GPT-4 is estimated to have over 1 trillion parameters. These breakthrough results are being tried and tested by people from all over the world across all sectors.
Among the key founders of deep learning, Ilya Sutskever is the co-founder and chief scientist of OpenAI and has continued to advance cutting-edge AI technology. However, Geoffrey Hinton recently left Google and has been advocating the idea that AI’s threat to humanity may be more urgent than climate change. Elon Musk, another co-founder of OpenAI, also called for a pause in the development and testing of language models more powerful than GPT-4. With all this, I can’t help but want to ask Feigenbaum, who is now 87 years old, one critical question. As the father of natural language processing AI, how should mankind take its next step as the era of strong AI approaches?